AI in healthcare revenue cycle: It's a thing, but it's complicated
- Jesse Ford
- May 16, 2023
- 4 min read
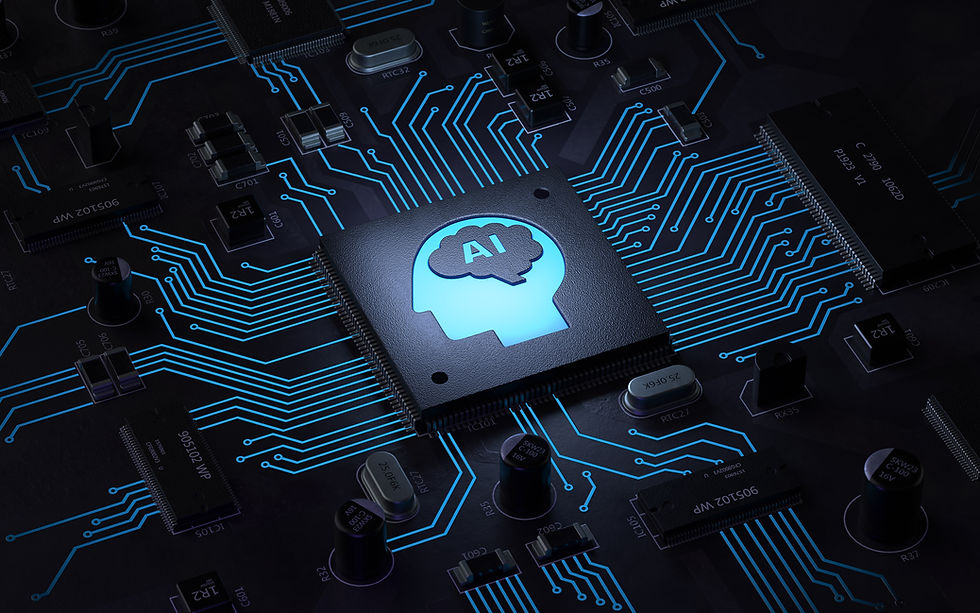
Like everyone else, I was spellbound by the sudden arrival of Chat-GPT from the Silicon Valley tech firm OpenAI. It seemed like a quantum leap in the evolution of computing, handling complex queries on any topic and within seconds turning the results into work product that would have taken a human days or weeks to accomplish. Not long thereafter came GPT-4, a marketable model that brings galactical amounts of data, code and images from the Internet and makes it available instantly for new applications, including editorial content, video animation and much more.
OpenAI didn’t invent new technology; it uses generative AI, a domain that came about in the middle of the past decade to produce various types of content. The brilliance of this chatbot is the simplicity of its user interface, the scale of its data and the decision to put out a version for anyone to use for free.
Whatever it is, ChatGPT generated a frenzy of activity from vendors new and old. Nobody wants to their brand to become next BlackBerry; everyone wants to be first to market with an AI-powered solution. The interest level of healthcare leaders in all things AI rose sharply, with nearly everyone plotting to implement applications by next year at the latest. Today’s staffing shortages and the skyrocketing cost of care seem ripe for the kind of disruption AI can bring.
For healthcare, machine learning and especially a subset called deep learning are more relevant tools. Deep learning involves running massive amounts of data through “webs” of mathematical formulations loosely inspired by the working of neural pathways in the human brain. As a network processes new data, connections between the parts of the network shift, building up an ability to interpret future events.
Two years ago, I wrote that in our world of revenue cycle, vendors were out over their skis in saying they had automated everything from clinical documentation to coding to claims management. I saw enough demonstrations of these solutions to know the early adoptions were basically pilot projects and not very good ones at that. A Google search using the terms “AI,” revenue cycle management” and “failure” yields ample proof of the many growing pains of this new digital domain. I also wrote that it was going to take about three years for this technology to mature into something that could begin to be integrated into business operations.
Two years later, much progress has been made, and we may still be on that timeline. In the meantime, we need to reset expectations of just what AI can and can’t do for healthcare revenue cycle management now and not overpromise its near future. There are still issues with Natural Language Processing (NLP), which can’t understand nuances of language. No matter how sophisticated, AI still lacks common-sense reasoning, and learning new skills from fewer than hundreds of thousands of examples.
Salud has always been technology-forward, having been founded to make revenue cycle management as productive, cost-effective and patient-centered as possible. For years we have used robotic process automation so staff can instantly check claims statuses and prepopulate payer forms. We have developed a proprietary screen scraper that automates the extraction of targeted information from the back end of payer websites and places it in a format that we can use in our dataset.
We have been building what we call a controller, an advanced software that launches our robotic processes automatically in the right sequence, so our digital queries are more productive and faster.
We are also using robust AI software to create a big-data infrastructure, feeding it massive amounts of data that will allow us to:
Identify potential problems such as problems with a patient’s insurance, incorrect codes and bills that don’t meet payer rules before a claim is filed
Estimate expected reimbursement and the patient’s share of the cost. This is what we call balance integrity; we want to be able to tell a patient the reason they have to pay a valid balance.
Predict claim denials by analyzing past claims by type or payer, using the insight to reduce recurring denials and improve workflows
We are frequently asked about computer-assisted coding (CAC), which uses NLP to highlight specific terms within documentation and then applies appropriate ICD-10 diagnosis and procedure codes. The advantage is you get a majority of codes already attached to accounts, allowing coding professionals to review for accuracy instead of having to manually enter codes or have providers entering them. While CAC can find patterns and anomalies in vast quantities of data, it is only as good as the NLP that feeds into it. Studies show that NLP still cannot identify some obviously incorrect data entries. CAC likely will get there, it’s just going to take a while.
Even with the most robust AI, you still need staff expertise, which is why Salud puts such an emphasis on continuous learning for our teams. What AI can do is vastly improve the information and analytics available to staff, making them problem solvers who are far more engaged and productive.
AI is getting closer to full functionality, but end-to-end automation of revenue cycle management is a long-term goal, not a current reality. And no matter how good AI gets, we humans will still know a few things machines can’t understand.
Contact us to find out how Salud's technology advantage can help you achieve your revenue cycle goals.